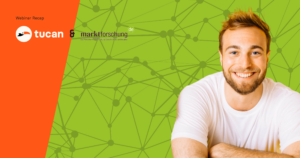
From data to insights: AI secrets for market research professionals
In an era where data is king, the integration of artificial intelligence (AI) into market research is revolutionizing the way we gather and interpret insights.
In an era where data is king, the integration of artificial intelligence (AI) into market research is revolutionizing the way we gather and interpret insights.
The GPT hype is causing a flood of new offerings for all markets. Sadly, very few are quality AI tools for market research. More often than not companies only build pretty front-ends for ChatGPT or utterly ignore data protection regulations. Tucan.ai can show you the possibilities of working with a real, sophisticated A.I. solution.
For us, 2023 is not only the year of language models – buzzword “ChatGPT” – but also the year of market research. Why? As our AI can automatically transcribe, code, summarize and smartly archive audio and video in several languages, it is particularly well suited for qualitative research.
Data may be more valuable than oil these days. But accumulating data just for the sake of data is useless, both scientifically and economically. To reach a better understanding of certain nuances and causalities it must be processed and analysed reliably. AI and natural language processing (NLP) come in handy here.
In 2023, AI is no longer a competitive advantage but a necessity. Those who can process large amounts of data, analyse metadata and recognise patterns will gain much deeper insights into customers’ minds. Others will fall behind. On the current state of AI in CX research.
As a market researcher or opinion miner, staying up-to-date with the latest industry trends and insights is crucial. One great way to do this is by listening to podcasts.