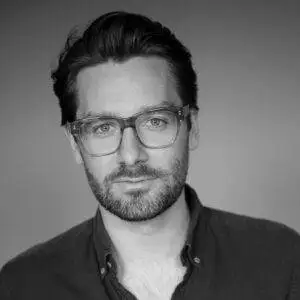
Lukas Rintelen
Co-founder & CEO
Tucan.ai
In our rapidly digitally transforming information age, it is becoming more and more difficult to deliver compelling and engaging customer experiences. Market leaders in virtually every industry are now putting personalisation at the heart of their business strategy.
For example, Rodney McMullen, CEO of Kroger, recently described seamlessness and personalisation as two very central areas in which the retail group is now primarily investing. Similarly, market leaders in the apparel (e.g. Nike), restaurant (e.g. Starbucks), banking (e.g. JPMorgan Chase) and home improvement (e.g. Home Depot) industries have all said in the past year that they are now strategically focusing on seamless, personalised omnichannel experiences.
Surveys show that customers who have had positive experiences with brands spend more on average and are more loyal in the long term. According to a study by Deloitte, they are willing to pay up to 140 percent more if they have had a positive experience with a brand.
Plus, we know that consumers of generations Y and Z predominantly prioritise brand experiences over products. That doesn’t necessarily mean they just want to go on holiday instead of spending their money on trendy sneakers: McKinsey documents a resurgence in demand for “real” shopping experiences.
Now the question is: What contribution can AI and ML make to optimising customer experiences, user retention and conversions?
Let’s first take a look at the current situation in market research in general before we go into more detail on how and for what machine and deep learning technologies can be used in CX research and which tools and providers are currently enjoying particular popularity.
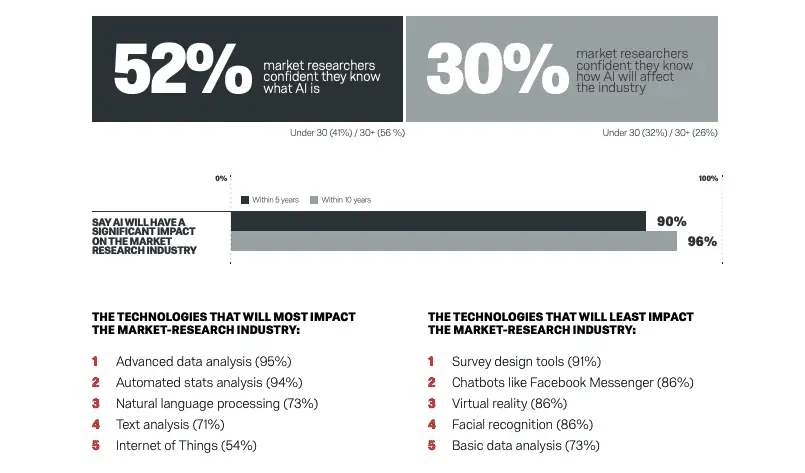
Use of AI grows rapidly across industries
We are now at a point where competitive advantage is increasingly derived from the ability to meticulously capture, analyse and process large volumes of customer data, and effectively use AI/ML to better understand, design and manage customer journeys.
To create impactful experiences, companies need ongoing insights based on a comprehensive, in-depth understanding of their customers. In digitalised, fast-moving markets, there is a need for innovative, agile and semi-automated CX research that combines diverse analytical methods and makes targeted use of AI/ML. Especially on this side of the Atlantic, however, the road ahead is still a rocky one for many.
In a variety of other industries, the use of AI/ML has already grown exponentially. An O’Reilly report from 2021 shows that IT and electronics tops the rankings (17%), followed by financial services (15%), healthcare (9%) and education (8%).
However, the fact that AI/ML still play a relatively small role in market research in no way diminishes the industry’s expectations:
According to a survey conducted by Qualtrics this year, a good half of the market research decision-makers surveyed are convinced that they know exactly what AI is; almost all of them assume that AI/ML will have a significant impact on the development of market research over the next ten years; and around 30 per cent are confident that they can adequately anticipate the impact. Trend researchers at the SAP subsidiary specialising in experience management also predict that in five years at the latest, at least 25 percent of all surveys will be conducted using digital assistants.
You want to test Tucan.ai for your Company?
Advantages for market and CX research
AI/ML tools help companies gain a better understanding of customer behaviour, what problems they have and how they react to certain offers. They make it possible to collect, analyse and interpret data more quickly and accurately.
At the latest since the publication of the language model ChatGPT developed by OpenAI a few weeks ago, computer linguistic methods for Natural Language Processing in particular have been the talk of the town. With NLP, text and speech data can be systematically collected and analysed to find out, for example, how customers behave and react to stimuli. Complex data patterns can then be recognised and interpreted better and better thanks to algorithms.
Long story short: AI/ML offers numerous opportunities to optimise collection and analysis processes. Despite the challenges of developing flexible models that can work with unpredictable language, the usefulness of using AI in market and CX research is unmatched. The following is an overview of the key benefits:
1. Saving resources
Arguably the biggest added value of AI/ML is the time it helps save. Effective automation can reduce the duration of a project from months to weeks or even days. As a result, analysts and marketers can spend more time evaluating, interpreting and telling the story behind the data than calculating generalisable numbers or trying to make sense of relevant wordings. AI/ML also allows companies to redeploy human resources elsewhere and reduce costs for external providers.
2. Higher data quality
There are many challenges that companies and brands face today when it comes to efficient access, use and exploitation of data. According to the latest Dell Digital Transformation Index (2020), data overload and “inability to gain insights from data” are the biggest barriers to successful digital transformation.
Also a major challenge in the area of data analytics are so-called data silos. This refers to data stores that can be accessed little or not at all, e.g. because they are poorly prepared or have been isolated in individual departments.
Although AI/ML are not a miracle cure for such silos, they offer companies tools to gain much deeper insights. Higher quality data usually contributes to much better results. After all, because AI/ML tools generally make it easier to analyse qualitative data by automating collection, processing and linking processes, we can now collect a lot more of it.
In addition, AI systems are now able to identify themes, correlations and subtle nuances in and between individual statements that are often overlooked and passed over in manual documentation. The free-text data obtained enables additional, usually deeper insights.
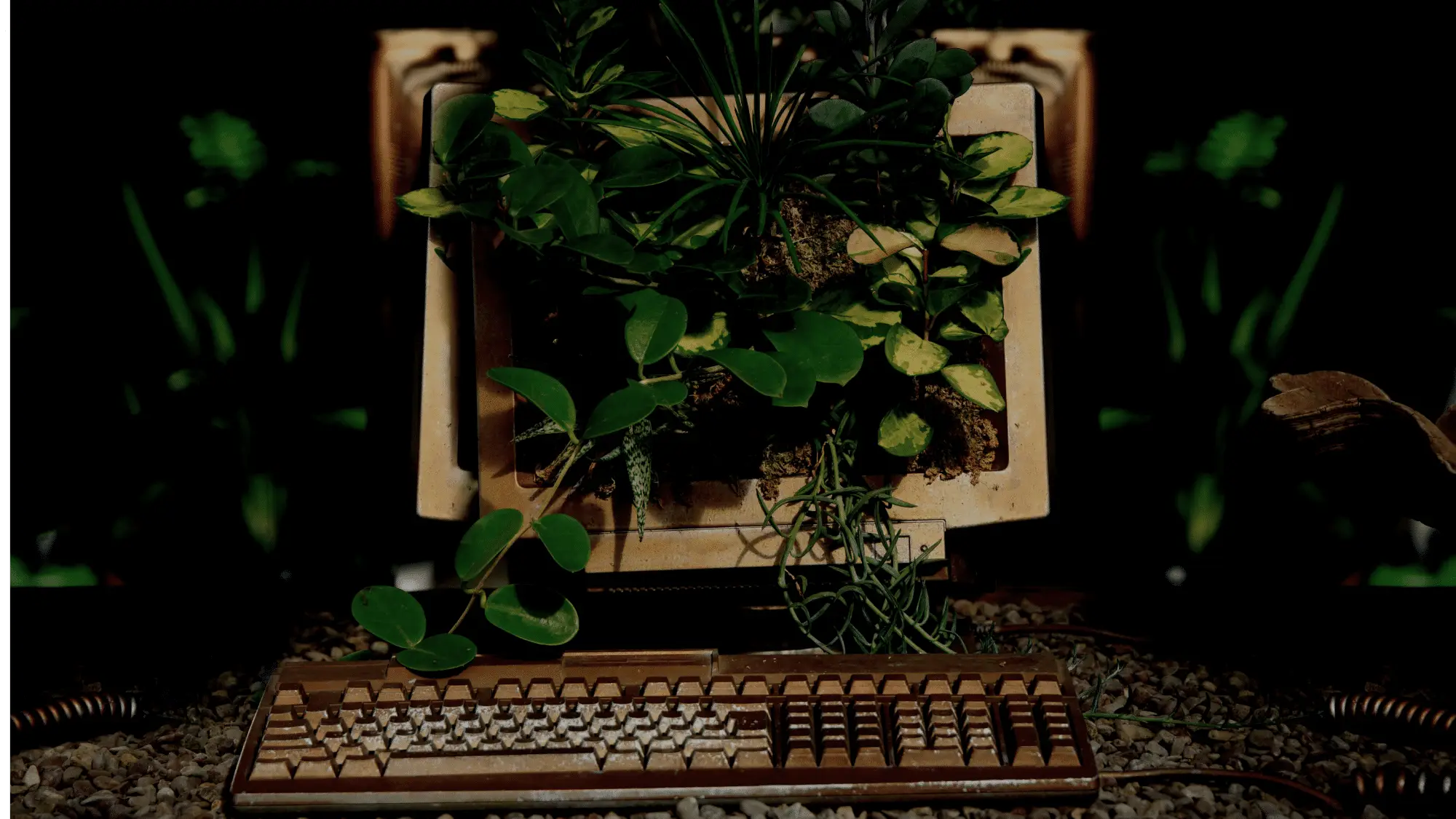
Use cases in CX research
In the following, I would like to present the most common use cases of AI/ML in customer experience analytics and a few innovative companies leading the way. The most widely used methods and tools include clustering and categorisation algorithms, text and sentiment analysis, and predictive models.
An absolute must-know for every market researcher and CX researcher is definitely Kantar Marketplace, launched in mid-2019. The automated platform, which is available in 70 countries, delivers decision-relevant insights in no time, whether companies need feedback on an idea, are developing a new product or launching a campaign. With Marketplace, Kantar offers an innovative toolbox that enables researchers, professionals and agencies to gain deeper insights through automation and make data-driven decisions faster.
1. Automatic collection
A promising way to drive CX enhancement, friction reduction and cost reduction go hand in hand is automation through so-called bots. In the new year, they are arguably the strongest and most visible trend towards AI/ML in market and customer research.
In response to the Corona pandemic, demand for AI assistants in the industry has skyrocketed. AI/ML-based assistance tools such as those from IBM, Zaoin and boost.ai help CX professionals automate and optimise analytics processes and customer relationships. Virtual agents, for example, pre-select customer queries and automatically answer routine queries. Using scripted rules, AI and ML, they generate automated responses, continuously learn new answers to basic questions through integrations with backend systems, and can handle a much larger volume of queries than humans.
2. Clustering algorithms
Speaking of big data: Most companies already capture a lot of customer interactions in their CRM systems. However, much of this data needs to be properly structured before it can actually provide insights. Using information about customer interactions, AI systems are now being trained to replicate the work of a customer service representative. Leading providers such as Qualtrics use clustering algorithms to do this.
Clustering is a technique that can automatically classify thousands of lines of unstructured conversations. In this way, a company’s customer service data can be retrieved for analysis in seconds and successful responses, frequencies, urgencies, etc. can be shown. Deep learning algorithms are used to automatically cluster data and elicit frequently asked customer questions and assess the extent to which support can be automated.
3. Automatic summarisation
AI also comes in handy for summarising videos and audios in CX research. Through the targeted use of NLP, we can now automatically transcribe and summarise gigantic amounts of video and audio feedback. Important information is thus captured more quickly and resources can be focused on more important tasks.
The German software company Tucan.ai, for example, offers AI-generated conversation summaries in addition to automatic recording, transcription and coding. This is particularly useful for those who want to analyse large amounts of data from interviews, group conversations, etc. and save time by not having to document or categorise them manually.
4. Categorisation and coding
The biggest challenge in analysing call data is usually the time and cost associated with manually coding and categorising collected data. Again, Tucan.ai’s NLP-based SaaS platform can provide a quick remedy. It is therefore already being used in this country by well-known industry players such as Kantar and GIM.
The Berlin-based deep-tech start-up has developed an in-house AI system that can, among other things, automatically code and categorise conversation data. Large amounts of data are thus processed faster and more precisely, allowing targeted and comprehensive analyses to be carried out and deeper insights into views and customer needs to be gained. This in turn enables key resources to be reallocated, recurring concerns to be responded to more quickly, and products and services to be adapted accordingly.
5. Predictive models
Link AI is an AI platform that predicts the performance of a digital advertisement in the market. It has been trained with Link, the world’s largest advertising database, which includes over 230,000 survey-based tests with 30 million real human interactions. Link AI breaks down each ad into individual images and further decomposes the content into images, audio, speech, objects, colours, text as well as other attributes. Then, using AI video processors, the machine extracts up to 20,000 features from the video file, feeds them into machine learning models and finally predicts the score of the ad based on metrics for “creative effectiveness”.
AI-driven predictive approaches also become increasingly popular for surveys. For example, SurveyMonkey uses AI-powered tools to help companies design and analyse surveys, predict completion rates and automate data analysis. The tool is widely used for customer and employee surveys, market research and other forms of feedback collection.
6. Audience Journey Tracking
Another area where computer learning is currently on the rise is audience journey mapping. With the help of AI, it’s now possible to precisely document a wide range of customer interactions with a brand or company from start to finish. This way, you can determine more precisely when customers experience friction losses or where exactly they deviate from the interaction process.
Well-known examples of AI-supported audience journey mapping include the web tools HotJar, Appier and Fullstory. They can be used, for example, to specifically observe individual website sessions of those customers who do not complete a purchase. This allows qualitative insights to be gained that are often not properly captured in statistical evaluations of large data sets.
For audience journey tracking, the smart software Knotch is becoming increasingly popular. It can bring together different interactions that customers have with companies over time and show those paths that bring the most conversions. Instead of analysing isolated campaigns that compete with each other for revenue share, Knotch accurately determines exactly how much impact each digital interaction or content had on sales.
7. Automatic sentiment analysis
Sentiment analysis uses AI and ML to determine the emotions expressed in words. It follows a predetermined metric to understand how positive, neutral or negative a statement or text sounds. AI systems can “pre-analyse” millions of comments on social media, rating portals and online surveys.
The innovative use of sentiment analysis outside of commercial market and customer analysis was demonstrated by the AI-powered social media analysis of En Marche in the course of the 2020 Senate elections in France: Emmanuel Macron’s party reportedly used NLP and sentiment analysis to gain deep insights into voters’ preferences expressed online on certain topics.
In CX research, it comes handy, in particular, for achieving the following goals:
Improving marketing campaigns: monitoring sentiments, understanding emotional responses to specific messages and better assessment of competitors’ reception.
Prioritisation in customer service: prioritise tickets faster, optimise queues through automation, enables faster, more specific responses to feedback
Detailed understanding of customers’ perceptions of products and brands
Trend analysis, forecasting: e.g. reactions to new interfaces or features
Churn prediction: e.g. capture negative sentiment online in real time
Sentiment analysis, often called opinion mining in English, is a sub-field of NLP. At its core, it automatically captures and categorises large numbers of individually expressed opinions, transforming unstructured data into actionable information. A real specialist for automated sentiment and emotion analyses, which Qualtrics Marketplace, among others, relies on, is, for example, the British start-up Adoreboard with its “Emotion AI Platform”.
From competitive advantage to necessity
Please note that this article does not claim to be exhaustive and merely aims to provide an initial overview of the myriad new players, tools and opportunities that have recently emerged in the CX segment thanks to rapid advances in AI/ML. There are numerous other great applications out there that deserve your attention as well. If you know of any that you would like to bring to our attention, we would of course love to hear from you.
Nevertheless, this article shows very clearly that the targeted use of AI/ML tools is no longer merely a promising competitive advantage, but has become a basic prerequisite for participating in the competition for attention and loyalty. Due to the increasing digitalisation of our society and the ever-growing competition in the online sector, it has become much more difficult to clearly stand out from competitors.
Those who can process large amounts of data, analyse metadata and recognise patterns in a short time gain deeper insights into the thoughts and behaviours of customers. Those companies that do not yet have this capability should start testing innovative solutions as soon as possible. Otherwise, they will inevitably fall behind in the coming years.